In our modern world, where data is as abundant as air, understanding how to extract valuable insights from it has become paramount. This is where analytics comes into play, offering various methodologies to make sense of the vast sea of data. But what exactly are these methodologies, and how do they differ? Let's embark on a journey to demystify four critical types of analytics: descriptive, diagnostic, predictive, and cognitive.
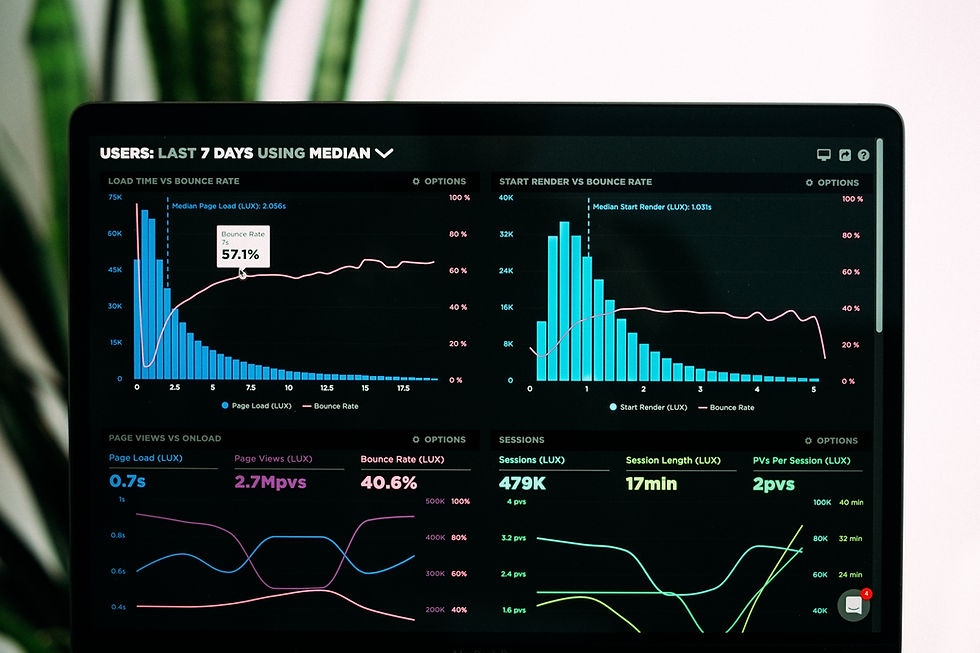
Descriptive Analytics: Painting a Picture
Imagine standing before a magnificent landscape, taking in every detail—the lush greenery, the rolling hills, and the serene lake. Descriptive analytics serves a similar purpose in data—it paints a vivid picture of what has happened. This form of analytics is like a snapshot of the past, providing summaries and visualizations that help stakeholders grasp current affairs.
Descriptive analytics answers questions like "What happened?" or "What is happening?" It's the foundation upon which further analysis is built, offering insights into trends, patterns, and anomalies within the data. For instance, a retail store might use descriptive analytics to understand its sales performance over the past quarter, visualizing data through graphs or charts for straightforward interpretation.
Diagnostic Analytics: Unveiling the Why
Once you've captured the scene with descriptive analytics, the next step is to investigate why things happened the way they did. This is where diagnostic analytics comes into play. It's akin to being an investigative journalist, unravelling the mysteries behind the data.
Diagnostic analytics seeks to identify the root causes of past events or trends. It helps answer questions like "Why did it happen?" or "What were the contributing factors?" Organizations can uncover insights that inform decision-making and drive improvements by conducting thorough analyses and investigating relationships between variables. For example, a healthcare provider might use diagnostic analytics to explore the reasons behind a sudden increase in patient readmissions, pinpointing potential gaps in care or treatment protocols.
Predictive Analytics: Peering into the Future
While descriptive and diagnostic analytics focus on the past and present, predictive analytics leaps into the future. It's akin to being a fortune-teller, using data to forecast potential outcomes or trends. Predictive analytics leverages statistical algorithms and machine learning techniques to make educated guesses about what might happen next.
This type of analytics addresses questions like "What is likely to happen?" or "What could happen if we take a certain action?" Predictive analytics enables organizations to anticipate future events and make proactive decisions by analysing historical data and identifying patterns. For instance, an e-commerce company might use predictive analytics to forecast customer demand for certain products, optimizing inventory management and marketing strategies accordingly.
Prescriptive Analytics: Providing Recommendations
Prescriptive analytics goes beyond predicting future outcomes by recommending actions to optimize those outcomes. It's like having a GPS navigator telling you where to go and suggesting the best route. By leveraging advanced algorithms and optimization techniques, prescriptive analytics helps organizations make data-driven decisions to achieve their objectives. For example, a manufacturing company might use prescriptive analytics to optimize production schedules and minimize operational costs.
Cognitive Analytics: Harnessing the Power of AI
As technology advances, so too does the field of analytics. Cognitive analytics represents the cutting edge, harnessing the power of artificial intelligence (AI) and machine learning to mimic human thought processes. It goes beyond traditional analytics by understanding data, learning from it, and making autonomous decisions.
Cognitive analytics encompasses various capabilities, including natural language processing, sentiment analysis, and image recognition. It enables organizations to extract insights from unstructured data sources such as text, audio, and video, unlocking valuable knowledge that was previously inaccessible. For example, a financial institution might use cognitive analytics to analyze customer feedback from social media channels, identifying emerging trends and sentiment shifts in real-time.
Conclusion
In conclusion, analytics is a multifaceted discipline with various methodologies suited to different purposes. From painting a picture of the past to peering into the future and harnessing the power of AI, each type of analytics offers unique insights that drive informed decision-making. By understanding the differences between descriptive, diagnostic, predictive, and cognitive analytics, organizations can unlock the full potential of their data and stay ahead in an increasingly competitive landscape.
Comments